With artificial intelligence (AI) applications growing rapidly across industries, organizations are under increasing pressure to manage and store enormous volumes of data efficiently. From training deep learning models to deploying real-time inferencing systems, AI workloads require fast, scalable, and cost-effective storage solutions. Maximizing data efficiency is critical not only for improving performance but also for managing infrastructure costs and environmental impact.
AI data storage isn’t one-size-fits-all. As use cases evolve—from computer vision and natural language processing to autonomous driving and fraud detection—the demands placed on storage architecture vary greatly. Efficiently managing both structured and unstructured data while ensuring rapid accessibility is a key priority for today’s data-driven enterprises.
Understanding the Unique Requirements of AI Workloads
AI workloads operate on massive datasets that often grow exponentially. These workloads are characterized by:
- High throughput: Training models can require transferring terabytes or even petabytes of data, demanding high-speed storage pipelines.
- Low latency: Real-time AI applications such as recommendation engines or autonomous systems must access data in microseconds.
- Diverse data types: AI applications process structured, semi-structured, and unstructured data types like text, video, and sensor data.
Designing an optimal storage system for these workloads means balancing performance, reliability, scalability, and cost.
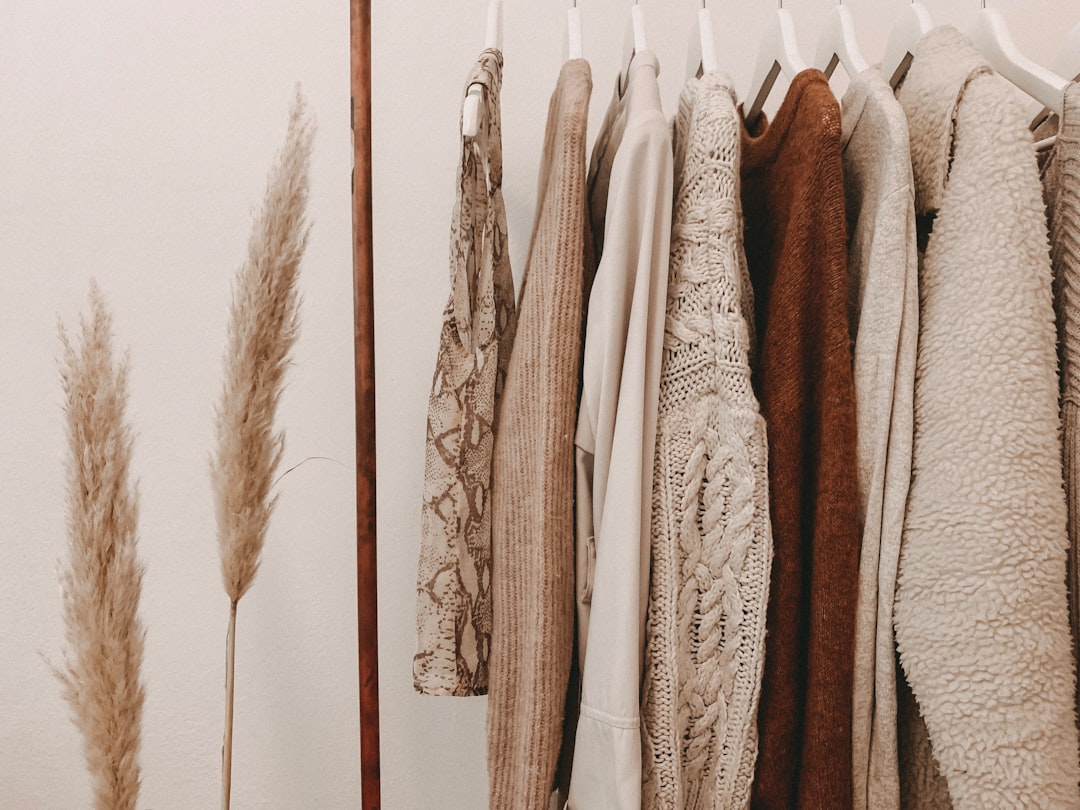
Key Storage Solutions for AI Efficiency
Multiple storage architectures can improve AI performance. Here are some of the most effective solutions:
1. NVMe-Based Flash Storage
Non-Volatile Memory Express (NVMe) flash storage is ideal for high-performance AI workloads. With significantly lower latency and higher input/output operations per second (IOPS) compared to traditional SSDs, NVMe speeds up data retrieval during model training and testing.
Benefits:
- Ultra-low latency for real-time analytics
- High bandwidth for data-intensive operations
- Scalable design ideal for distributed AI workloads
2. Object Storage
AI systems often work with unstructured data like images, videos, and logs. Object storage offers scalability and metadata-rich architecture, making it a popular choice for long-term data storage and feeding large training datasets.
Benefits:
- Massive scalability for petabyte-scale data
- Cost-effective long-term storage
- Improved data management with metadata tagging
3. Hybrid Cloud Storage
By combining on-premises infrastructure with cloud-based resources, hybrid cloud storage offers the flexibility needed to handle dynamic AI workloads.
Benefits:
- Operational flexibility—burst to the cloud during high demand
- Centralized management across environments
- Integration with cloud-native AI tools and services
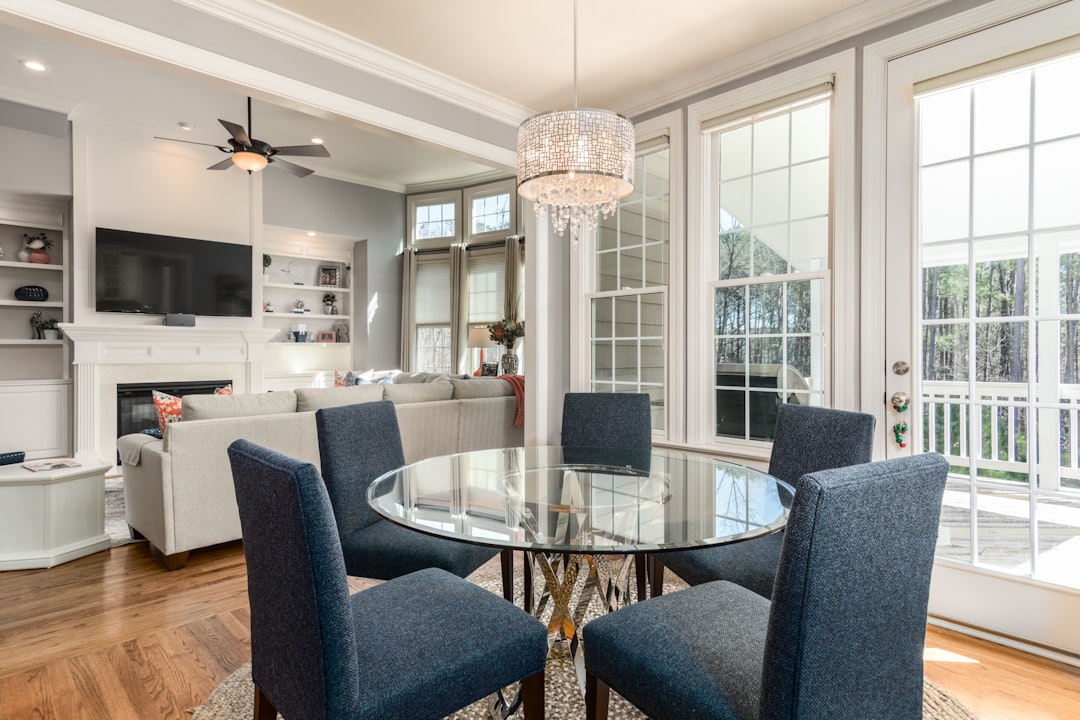
Best Practices for Maximizing AI Data Efficiency
Simply choosing a storage solution isn’t enough—you need to adopt strategies that ensure long-term efficiency and performance. Here’s how to make the most of your storage infrastructure:
- Implement tiered storage: Store frequently accessed data on high-performance media like NVMe, while archiving older data on lower-cost storage.
- Use data pre-processing pipelines: Reduce storage load by cleaning and transforming datasets before feeding them into models.
- Leverage intelligent data caching: Minimize latency by storing active datasets closer to the processing units using in-memory storage or AI-specific caches.
- Monitor and scale: Use analytics tools to track storage usage and performance to anticipate scaling needs efficiently.
Looking Ahead
AI technologies are becoming more advanced, and so are the data demands. Edge AI, generative models, and continual learning systems will only accelerate data growth. Investing in scalable and efficient storage solutions is no longer optional—it’s a prerequisite for success in the AI-driven world.
By harnessing the right mix of storage technologies and operational strategies, organizations can not only improve AI model performance but also gain agility, reduce costs, and support sustainable growth in the era of big data.
Choosing the correct storage architecture is just one step. Adapting continuously to evolving AI needs will define the new frontier in data intelligence and innovation.